Metabolomic data analysis is a specialized field of bioinformatics and data analysis that focuses on the study of metabolites within biological systems. Metabolites are small molecules, such as sugars, amino acids, and lipids, that serve as the end products of cellular processes and provide a snapshot of an organism's biochemical state. The goal of metabolomic data analysis is to understand the complex interplay of these molecules within a biological system, whether it's a cell, tissue, organism, or even an ecosystem.
Creative Proteomics provide metabolomic data analysis service. Our services go beyond mere analysis; we offer a holistic approach that includes data integration, stringent quality control, customization, and robust data reporting. We understand that each research project is unique, and we are dedicated to tailoring our services to meet your specific objectives. Whether you're delving into metabolic pathways, seeking biomarkers, or conducting time series analyses, our versatile solutions empower you to uncover the hidden secrets within your metabolomic data.
We leverage cutting-edge bioinformatics software and adhere to the highest quality standards, ensuring that the results are both accurate and reliable. We support a wide range of sample types and maintain compliance with regulatory standards for the secure handling of sensitive data.
Workflow of Metabolomic Data Analysis Services
1. Sample Collection and Preparation
Our process begins with the collection of biological samples, including tissues, blood, urine, and more. We employ meticulous sample preparation techniques to ensure the isolation of metabolites while removing unwanted contaminants, ensuring the integrity of your samples.
2. Mass Spectrometry Data Acquisition
Utilizing high-throughput mass spectrometry instruments, such as liquid chromatography-mass spectrometry (LC-MS) and gas chromatography-mass spectrometry (GC-MS), we perform precise measurements of metabolites. This technology provides detailed information about the molecular weight and structure of metabolites.
3. Data Preprocessing
Raw data obtained from mass spectrometry instruments are often noisy and contain variations. Our data preprocessing techniques, including baseline correction, normalization, and filtering, ensure the reliability and quality of your data for further analysis.
4. Metabolite Identification
Identifying detected metabolites is a crucial step. Our expert team employs bioinformatics tools and databases to match experimental data to known metabolites, utilizing spectral matching, accurate mass matching, and fragmentation pattern analysis for accurate identification.
5. Quantification
Following identification, we quantify the concentration of each identified metabolite in your samples. This step is essential for understanding the relative abundances of metabolites and their biological significance.
6. Statistical Analysis
Our statistical analysis techniques help identify differences between sample groups and highlight metabolites with significant changes. We provide comprehensive statistical analysis to enable you to draw meaningful conclusions and identify potential biomarkers or key metabolites of interest.
7. Pathway and Network Analysis
To provide a holistic view, we integrate your metabolomic data with other omics data, such as genomics and proteomics. Pathway analysis tools are used to interpret how changes in metabolite levels impact biological processes, allowing you to gain deeper insights into the biological context.
8. Data Visualization
Our tools for visualizing data, which encompass heatmaps, scatterplots, and pathway maps, serve the purpose of rendering results in a visually comprehensible manner. These visual representations are instrumental in simplifying the communication of intricate discoveries and patterns.
9. Validation and Interpretation
Finally, we ensure the validity of your results through additional experiments or cross-referencing with existing knowledge. Our experts help you interpret the data within a biological context.
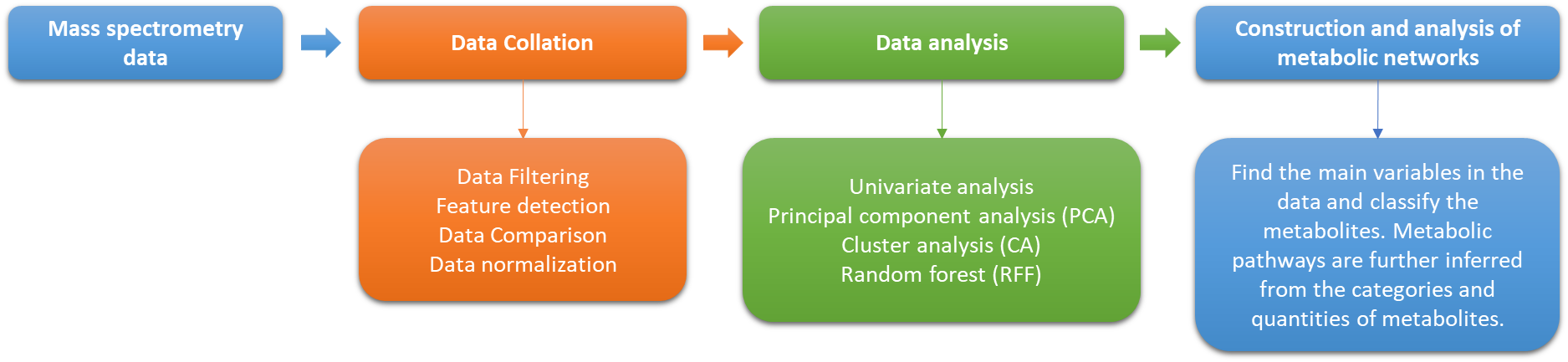
Metabolomic Data Analysis Provided by Creative Proteomics
Basic Metabolomic Data Analysis
Analysis Type | Description |
---|
Data Preprocessing | - Data normalization and scaling - Handling missing values - Data transformation (e.g., log transformation) - Batch correction (if applicable) |
Univariate Analysis | - Identification of significant differences between sample groups - Common tests: t-tests, ANOVA, Wilcoxon tests, etc. - Visualization with box plots and volcano plots |
Multivariate Analysis | - Exploring patterns within the entire dataset - Methods: Principal Component Analysis (PCA), Partial Least Squares-Discriminant Analysis (PLS-DA), Orthogonal Partial Least Squares-Discriminant Analysis (OPLS-DA) |
Pathway Analysis | - Identifying metabolic pathways affected by changes in metabolites - Assessing pathway enrichment and impact on cellular processes |
Advanced Metabolomic Data Analysis
Analysis Type | Description |
---|
Feature Selection | - Identifying relevant metabolites for classification or regression models - Methods: Recursive Feature Elimination (RFE), L1 regularization (Lasso), etc. |
Clustering Analysis | - Grouping samples with similar metabolite profiles - Methods: Hierarchical clustering, k-means clustering, etc. |
Time Series Analysis | - Investigating changes in metabolite profiles over time - Visualizing trends and patterns through time course analysis |
Network Analysis | - Constructing metabolic networks from metabolite data - Identifying key metabolites and their interactions within the network |
Correlation and Regression | - Assessing relationships between metabolites and external factors (e.g., clinical variables) - Linear and nonlinear regression, partial correlation analysis, etc. |
Machine Learning | - Building predictive models for classification and regression tasks - Methods: Support Vector Machines (SVM), Random Forest, Neural Networks, etc. |
Pathway Impact Analysis | - Evaluating the impact of metabolite changes on specific pathways - Assessing the biological relevance of detected metabolites in the context of pathways |
Metabolite Set Enrichment | - Identifying sets of metabolites that are significantly altered in specific conditions or pathways - Assessing enrichment of metabolite sets for biological insights |
Biomarker Discovery | - Identifying specific metabolites as potential biomarkers for diseases or conditions - Receiver Operating Characteristic (ROC) analysis, cross-validation, etc. |
1. Q: How to do further screening for differential metabolites?
A: Screening for differential metabolites is generally performed using a combination of thresholds such as P-value < 0.05 vip=" ">1.
P-values are derived from unidimensional statistical analysis (e.g., T-test). VIP values are derived from multivariate statistical analysis (e.g., OPLS-DA) characterizing the value of the contribution of that variable to the difference between the two groups.
There is also a practice of using P-values <0.05 logfc="">1 or logFC<-1) [FC=Fold change] for differential screening, but both P-values and FC values are sourced from univariate statistical analysis.
To do further screening on this basis, there are several approaches.
(1) P-value < 0.05, ranking the VIP values (the larger the VIP value, the more significant the differential metabolite).
(2) VIP>1, to rank the P values (the smaller the P value, the more significant the differential metabolite).
(3) Within the range of P values <0.05 vip=" ">1, do ranking on logFC values (the larger the logFC greater than 1, the more significant; the smaller the logFC less than -1, the more significant).
Restricted to stricter base screening conditions, e.g., p-value <0.01& vip=" ">2.
2. Q: What to do if you can't find differential metabolites during screening?
If common screening criteria (VIP > 1 and P < 0.05) don't yield any differential metabolites, you can start by widening the threshold, such as VIP > 1 and P < 0.1. If you still can't identify differential metabolites, you can perform univariate statistical analysis on the detected substances, using criteria like FC > 1.5 and P < 0.05. This analysis compares the expression values of individual metabolites among different sample groups and visualizes the differences using a volcano plot.
3. Q: Once you've identified differential metabolites, how do you verify their functions?
First, you need to conduct qualitative and quantitative validation. This typically involves using triple quadrupole mass spectrometry for targeted validation to obtain absolute quantitative information. Additionally, based on preliminary metabolic pathway analysis, if you know which regulatory pathway the differential metabolite belongs to, you can perform functional validation on the molecules within that pathway. Furthermore, you can complement the analysis by incorporating data from other omics approaches such as transcriptomics and proteomics.
4. Q: What is the volcano chart and what is it for?
A: The volcano map mainly shows the information of two dimensions, P-value and Fold-Change. All these information is closely related to the screening of differential metabolites, so it shows the distribution profile of differential metabolites in all substances.
5. Q: What is a Venn diagram? What is the use of it?
A: A Venn diagram is used to show all possible logical relationships between a finite set of different.
6. Q: What do R2 and Q2 mean respectively?
A: R2X (for PCA) or R2Y (for PLS-DA) indicates the proportion of the data variance or variance that can be explained by the current model, indicating the goodness of fit (the fit). Q2 indicates the proportion of the data variance that can be predicted by the current model, i.e., the prediction rate, indicating the predictive power of the current model.
7. Q: What is the difference between PLS-DA and OPLS-DA models?
A: OPLS-DA has an additional positive exchange calculation than PLS-DA, which filters out signals that are not relevant to the model classification. For example, if the difference between groups is relatively small and the difference within groups is relatively large, the PLS-DA VIP is used to screen out what may be the within-group difference variable, which is easily misleading. OPLS-DA is an upgraded version of PLS-DA.
8. Q: Permutation test judgment criteria?
A: The replacement test is the randomization test or re-randomization test. The usual criteria are R2<0.3 and Q2<0.05, but sometimes the sample biology is too small to meet the requirements, so only the slope of the regression line needs to be positive.
9. Q: Why is the comparative analysis only two by two?
A: Because in the search for differentials it is determined whether the substance is a differential based on the difference in content. The amount of a substance in one group rises/declines relative to the other group, while the amount of change relative to the other two groups cannot be calculated at the same time.
10. Q: Can the sample sizes be different for the two comparison groups?
A: Yes, only the minimum number of biological replicates in each group is required to be met.
11. Q: Can data from multiple platforms be integrated into one piece for PCA?
A: Yes.
12. Q: Is internal standard added in untargeted metabolomics LC-MS? What is its specific role?
A: Internal standard (2-chloro-L-phenylalanine) is indeed added in untargeted metabolomics, but it does not participate in any data analysis. It is solely used by the laboratory for internal assessment of instrument and experimental stability.
13. Q: For blood samples undergoing untargeted metabolomics analysis, which is better, serum or plasma samples?
A: Both serum and plasma are samples obtained after processing blood, and existing literature reports differences in the types and abundance of metabolites between serum and plasma. However, for research purposes, there is no clear indication that one sample type is superior to the other. Therefore, when choosing between serum or plasma, it is only necessary to ensure uniformity at the time of collection, and blood samples are preferably collected with EDTA or heparin anticoagulants. During collection, hemolysis should be avoided, and samples should be stored at -80°C to prevent repeated freeze-thaw cycles.
14. Q: Does repeated freeze-thaw cycles significantly affect metabolite detection?
A: Studies have shown that freeze-thaw cycles can cause changes in metabolites, and analysis of these substances reveals no intersection between differential substances. Therefore, differential substances selected using frozen-thawed samples likely include differences caused by freezing and thawing. In other words, freeze-thaw cycles can generate new differential substances, resulting in inaccurate representation of the true metabolic levels of the samples.
15. Q: How many substances can be detected in untargeted metabolomics?
A: Nanomix Metabolomics' comprehensive targeted metabolomics database contains over 5000 metabolites. All detected metabolites through LC-MS/MS analysis platform must undergo primary and secondary matching with metabolites in the standard library. This standard library data includes amino acids and derivatives, nucleotides and derivatives, flavonoids, terpenes, phenylamines, fatty acids, etc.
16. Q: Why can't some common metabolites be detected in untargeted metabolomics?
A: Firstly, untargeted metabolomics detection is not targeted towards specific metabolite types of interest, so detected metabolites may not be the desired results. Secondly, the untargeted metabolomics detection technology may interfere with metabolites with low signal intensity, leading to signal masking and difficulty in identifying the substances of interest. Additionally, the detection range should be considered; Nanomix Metabolomics' untargeted metabolomics mass spectrometry scanning range is 100-1000 m/z, and substances whose mass-to-charge ratio falls outside this range cannot be detected. If there is a specific research direction, it is recommended that customers use targeted metabolomics. Targeted metabolomics focuses on the detection of specific metabolites of interest, and the detection results are more ideal.
17. Q: What do "pos" and "neg" mean in untargeted metabolomics results? How are these two types of metabolites treated during analysis?
A: "Pos" and "neg" represent positive ion and negative ion modes during data acquisition. Positive ion and negative ion modes are two sets of mode data generated during data acquisition, so primary analysis (MS) results provide lists of detection results for both pos and neg modes. However, in secondary analysis (MS/MS) results, we merge the substances identified in positive and negative ion modes, so secondary analysis is done based on metabolites and does not distinguish between positive and negative ion modes.
18. Q: Why are there thousands of feature peaks detected in untargeted metabolomics results, but very few compounds are finally identified?
A: Currently, metabolomics typically identifies around 300-400 metabolites. Data analysis uses strict standard databases for comparison, with low false positives. Some metabolites may not be in the standard database, so they cannot be detected; public databases match based solely on molecular weight, resulting in many candidates but high false positives. Additionally, a metabolite may be detected multiple times in different forms of ions (charged), such as protonation, deprotonation, adduct ions, isotopic peaks, dimers, trimers, and unique ion forms, so there are many detected ion peaks, but many can only be qualitatively identified as one metabolite.